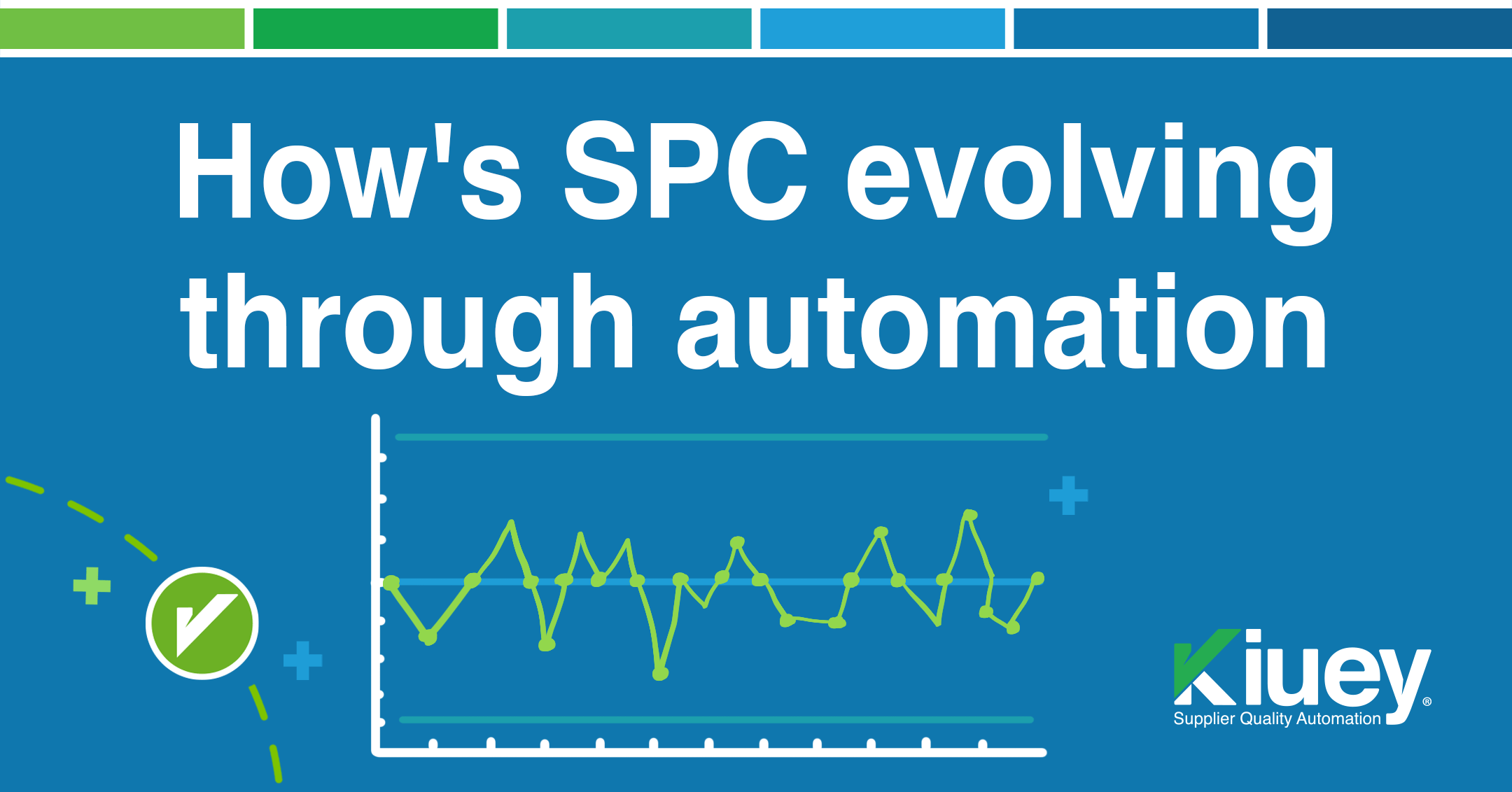
October 25, 2024
Statistical Process Control (SPC) has been a cornerstone of quality management for decades. It relies on the principle of sampling to estimate population parameters and monitor process stability. However, the advent of advanced technologies has fundamentally changed the way we collect and analyze process data. With the ability to capture every data point, the traditional concept of sampling is being challenged. This article explores how to effectively leverage automated data to enhance process control and decision-making.
The Evolution of Data Collection
In the past, collecting process data was a labor-intensive and time-consuming task. Quality engineers relied on manual sampling to gather representative data points. However, modern manufacturing systems are equipped with sensors and automated data collection systems, enabling the capture of vast amounts of data in real-time.
Challenges and Opportunities of Automated Data
While automated data offers numerous benefits, it also presents unique challenges:
- Over-reliance on Data: The abundance of data can lead to over-analysis and unnecessary adjustments. It’s crucial to focus on meaningful insights and avoid reacting to minor fluctuations.
- Measurement System Variability: The accuracy and precision of measurement systems must be rigorously assessed and maintained to ensure data reliability.
- Data Integration and Analysis: Integrating data from various sources and analyzing it effectively requires robust data management and analytics tools.
Key Strategies for Effective Process Control with Automated Data
- Establish Robust Measurement Systems:
- Calibration and Verification: Regularly calibrate and verify measurement systems to minimize measurement error.
- System Suitability Testing: Implement a systematic approach to assess the performance of measurement systems over time.
- Implement Data-Driven Decision Making:
- Statistical Process Analysis (SPA): Utilize advanced statistical techniques to identify trends, patterns, and anomalies in process data.
- Control Charts: Adapt control charts to continuous data streams, such as using moving range charts or exponentially weighted moving average (EWMA) charts.
- Predictive Analytics: Employ predictive modeling to forecast potential issues and proactively take corrective actions.
- Optimize Data Collection and Analysis:
- Rational Subgrouping: Carefully consider the appropriate sampling frequency and subgroup size to balance data granularity and computational efficiency.
- Data Quality Assurance: Implement data quality checks to ensure data integrity and accuracy.
- Data Visualization: Use effective data visualization techniques to communicate insights clearly and concisely.
- Foster a Culture of Continuous Improvement:
- Empowerment: Empower employees to identify and solve problems using data-driven approaches.
- Collaboration: Encourage cross-functional collaboration to share knowledge and best practices.
- Learning from Failures: Analyze root causes of process failures and implement corrective actions to prevent recurrence.
Conclusion
Automated data has the potential to revolutionize process control by providing unprecedented insights into process behavior. By embracing these strategies, quality engineers can harness the power of data to optimize processes, reduce defects, and improve overall product quality. However, it’s essential to strike a balance between leveraging data and avoiding over-reliance on it. A thoughtful and strategic approach is key to maximizing the benefits of automated data while minimizing the risks.
Let's talk to see how PPAP Manager can help your company to save time and money.